AI is not an ominous force to be feared, but rather a resource that should be harnessed cautiously
The key lies in understanding that AI is not an ominous force to be feared, but rather a resource that should be harnessed cautiously. It's imperative to strike a balance between acknowledging the technology's challenges and recognising its merits. By avoiding sensationalised hype and instead focusing on the specific areas where AI can truly shine, the aviation community can ensure that the benefits far outweigh any drawbacks.
What Does AI and Machine Learning Mean at NATS?
Machine Learning is a branch of AI which involves the utilisation of extensive historical data and information to train and educate machines, enabling them to formulate predictions, anticipate future outcomes and identify patterns. This process revolves around the machine comprehending past trends and extending that knowledge forward onto new data, which can quickly provide insight. Interestingly, Machine Learning may be either supervised or unsupervised, with the latter primarily used to identify aspects which may not be obvious to a human when interrogating large datasets.
AI is much broader, involving machines performing tasks that would typically have required human intelligence to accomplish. This often combines Machine Learning with text inputs from multiple sources, speech, reasoning ability, adaptive responses, image recognition, vision and/or physical movement to create ever more sophisticated and diverse tools. AI therefore refines and augments its capabilities by drawing on a continual spectrum of information, thus evolving its problem-solving prowess.
AI refines and augments its capabilities by drawing on a continual spectrum of information, thus evolving its problem-solving prowess.
Where Does NATS Utilise AI and Machine Learning Already?
Our Analytics team has been working with AI models to aid in the modernisation of UK airspace. Across the organisation, Analytics is helping establish where it is best to utilise AI to tackle complex problems, as well as situations where AI is not an appropriate solution.
Within the commercial space, one key area that NATS utilises AI and Machine Learning is through Demand Capacity Balancer (DCB). DCB prioritises safety and efficiency and complements existing Air Traffic Flow Management systems. DCB acts as a digital twin to deliver fast-time simulation of an airport; ingesting data from trusted sources and providing a rapid simulation of multiple scenarios so that operational outcomes and data can be used to proactively plan both in real-time and up to six months in advance. This assists heavily in collaborative decision making, as well as mitigating airborne and ground delays which in turn improves the passenger experience and decreases CO2 emissions.
AI is also utilised across the corporate areas of the organisation to assist and streamline processes. For example, AI modules used within regular reporting tools translate data into reports with automatic Natural Language summary for customers. This provides end-users with clear and timely updates, also reducing the requirement for analyst time in providing the interpretation.
Another example of Machine Learning being implemented within NATS is the evolution of air traffic forecasting algorithms. Since launching earlier this year, the short-term and medium-term traffic forecasts now use Machine Learning models to predict which flights will or won’t enter UK airspace, with an impressive average accuracy of over 95%, these traffic forecasts are clearly important to various teams across NATS, including helping ensure the operational staffing matches the predicted traffic volumes.
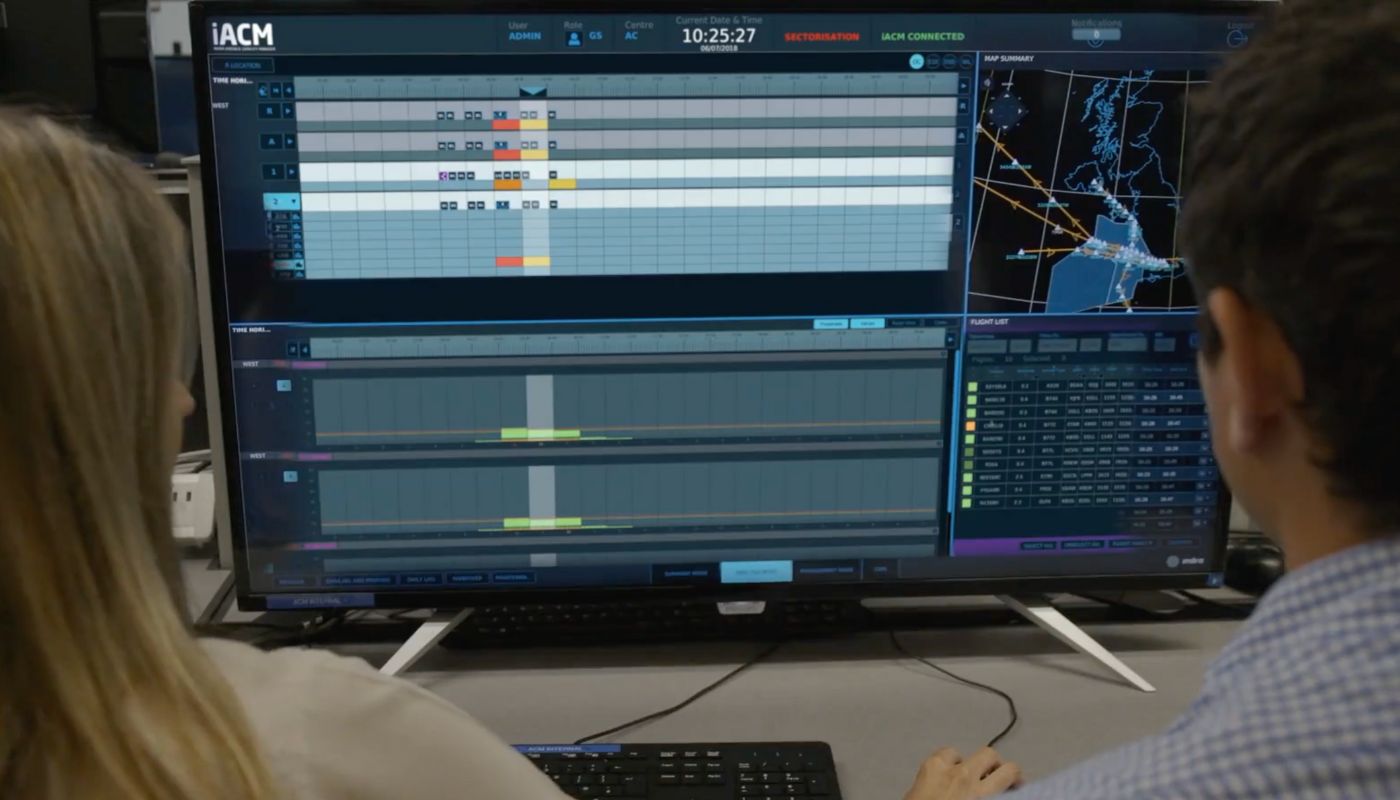
NATS is using machine learning to help predict traffic volumes.
As well as assisting operational outcomes, NATS teams have utilised elements of AI and Machine Learning to support employees and workload performance rates. In 2019, our Human Factors and Analytics teams developed Michelangelo, a tool to predict and manage the cognitive workload for operational staff. If workload is too high or too low, the efficiency and safety of the operation could be compromised. Michelangelo is built on anonymised workload data collected in operations, using the controller responses from workload surveys to match to systems data.
The impact of projects and changes are forecasted in terms of five metrics: controller workload, enabled capacity, safety, service (i.e. delays) and environment. Crucially, this tool determines contributing factors and the relationship between each of these metrics. The workload score links all metrics together, with a holistic view of workload provided in a standardised way, enabling optimal decisions in terms of balancing the effect of potential changes for both operational workload and for the impact on future air travel.
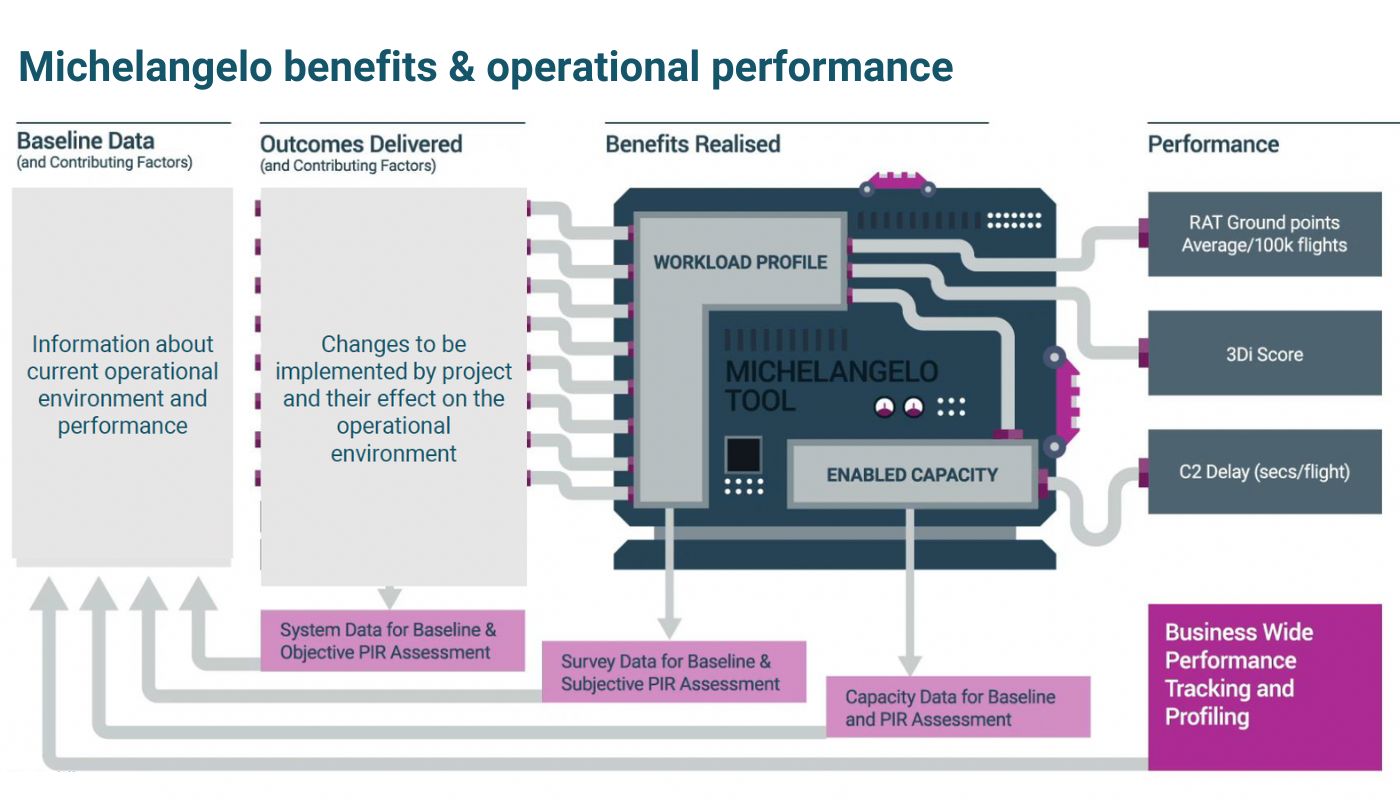
Michelangelo uses AI to predict the workload of operational staff.
Michelangelo is just one example of how NATS is using AI to assess data holistically and pinpoint potential improvements. Using both supervised and unsupervised learning techniques in a holistic way is an active area for Analytics and has proved to help identify relationships between variables in data sets, including those which wouldn’t typically come to mind when considering what might affect aspects such as the environmental efficiency of flights.
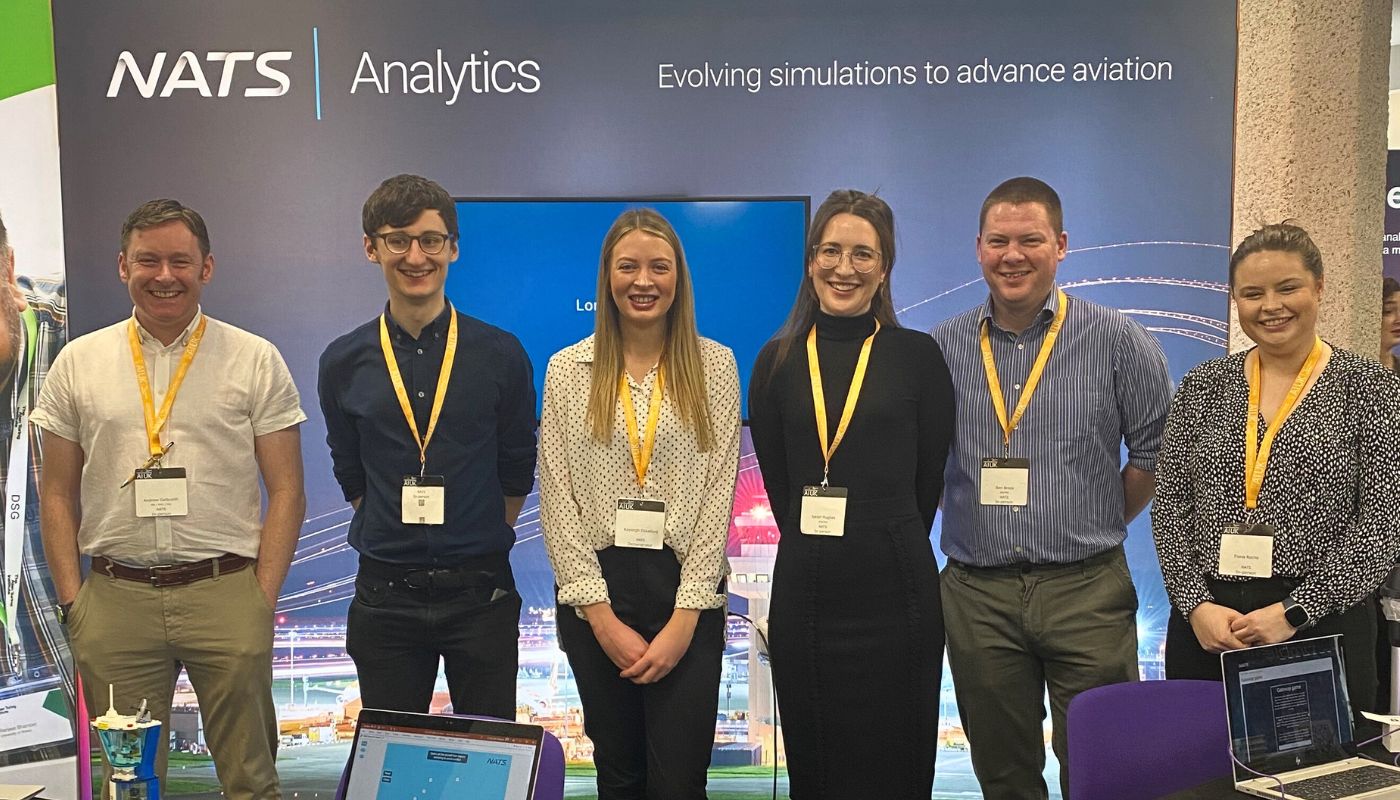
Some of the Analytics team discussing AI at a recent STEM event.
Analytics’ work with AI has created a strong foundation for NATS to navigate the ever expanding horizons of technology, whilst continuing to keep the skies safe. As this work develops and continues to integrate into daily processes, even more opportunities will arise where human innovation can harmonise with technology, ensuring a future that thrives on the strengths of both.